Data mesh: Rethinking the data architecture
Written By: (Eraj Mehmood – AVP, Abid Shafiq - Principal consultant Ammar Afzal – Junior Consultant, Data Analytics
March 22, 2022
Inherent to the growing use of big data, data warehouses, and data lakes are concepts that are already well established and implemented by numerous organizations to serve their decision-making needs. The monolithic architectural structure of today's data platforms has proven to be a significant roadblock for many. Centralized data architectures have certain limitations in delivering with speed and resilience.
After analyzing the various limitations demonstrated by monolithic architectures, the newest data architecture, "Data Mesh," can solve traditional bottlenecks and existing limitations. Data mesh consists of implementing an architecture where data is intentionally distributed among several mesh nodes to avoid data chaos or silos.
Demystifying data mesh
The term "Data Mesh" was first coined by Zhamak Dehghani from ThoughtWorks, which defines data mesh as "a socio-technical shift — a new approach to collecting, managing, and sharing data for analytical purposes."
We can say, that data mesh is an exciting new approach to designing and developing data architectures. Its design pattern breaks giant, monolithic enterprise data architectures into subsystems and domains. Data mesh's decentralized strategy distributes data ownership to domain-specific teams and serves data-as-a-product. The main objective of data mesh is to eliminate the challenges related to the availability and accessibility of data from its sources.
The 4 pillars of the data mesh architecture
Domain-oriented decentralized data ownership
Data mesh architecture is aimed to deliver the decentralized responsibility of data distribution to support scalability. In data mesh, data is broken down around specific business domains. Under this model, each business domain (sales, finance, customer services, HR, etc.) takes data ownership. Moreover, data mesh helps avoid data silos by adopting the concept of "data as a product" for each domain function.
Data-as-a-product
This means applying widely used "product-thinking" around the data, making the data a first-class citizen with features to eliminate particular needs. Data is discoverable and readily available; likewise, customers buy products using e-commerce platforms. The concept of "data as a product" ensures basic functionalities like discoverability, addressability, data trustworthiness, self-describing semantics, interoperable standards, and data security with data.
Self-service data infrastructure as a platform
Self-serve data infrastructure means providing a rock-solid foundation, unified tools, and interfaces for diverse domain users. Due to self-service data platforms, the infrastructure and platforms allow diverse domain owners to manage their data efficiently through integrated tools. However, business domains can make provisions like schemas, lineage, and attributes like data locality.
Federated computational governance
A data mesh implementation demands a robust governance model that embraces decentralized domain sovereignty, global standards, and automated executions for decision-making. This is made possible by a federated computational governance framework and policies. This governance model is a significant bridge to keep the right balance between centralized and decentralized data environments.
Why should organizations think about data mesh architecture?
According to Statista, global data generation will exceed 180 zettabytes in 2025. The existing data platforms have architectural limitations that hinder enterprises' ability to process data at scale. At its core, data mesh is designed to solve the problem of scaling. Here are some reasons your organization should adopt a data mesh architecture.
Centralized or monolithic data models may no longer be efficient
Centralized distributed systems sometimes face data silos, inconsistency, and a deficit in data integration. A data mesh architecture addresses this problem by decoupling and decomposing the centralized architecture, resulting in more effective ETL jobs.
More accessibility to data
Without a doubt, data accessibility is one of the most significant advantages of the data mesh architecture. This framework enables business owners to be in charge of their data. This way, the team and business owners can transform and integrate data for enhanced business value.
Standardized data observability
As enterprises view standardized data discoverability and observability as a valuable layers within their data architecture, data mesh ensures data observability. It optimizes data health on a best-practice scale, making this an essential part of its entire strategy.
Enhanced scalability and speed
Organizations have observed more speed and scalability after implementing a robust data mesh architecture. Because data is directly available, there is less dependency on data and business intelligence teams to manually update the data. This allows teams to configure and provision data with more scalability.
Data mesh: Understanding & addressing limitations
Data mesh might offer scalable data delivery with room to grow and be more resilient than other architectures. However, it doesn't mean a perfect fit for every organization. There are a few challenges even with a decentralized structure and computational governance. Furthermore, inherent complexities arise when data is managed across multiple autonomous domains.
Here are the top 3 challenges that the existing data mesh approach faces.
Data ingestion and duplication
Setting up the data ingestion framework is one of the most challenging aspects of data mesh architecture. Sometimes, data ingestion & configuration is required repetitively. This straying of data leads to redundancy, inconsistency, and duplications. Further, the duplication might lead to inefficiencies, impacting both the enterprise data ecosystem and the increased cost of ownership.
The process of adoption is slow
Most organizations still prefer traditional data management approaches; that's why data mesh adoption is relatively slow. Decentralizing the entire organizational data is not a quick step; it is time-intensive and needs 360-degree infra-upgradations. Organizations should be ready to put much effort before implementing a data mesh architecture.
Cross-domain analytics
While the data mesh architecture eliminates many data glitches, it fails to meet the critical functionalities offered by the data. Cross-domain analytics is one of those. The data mesh architecture must be standardized in formatting, discoverability, and other data features to address this limitation in cross-domain collaborations.
Key takeaways
Data mesh, in many ways, represents an entirely new approach to data management. While it certainly answers how the new data architecture is made to leverage data ecosystems, perhaps the most significant implementation challenge is to address the cultural change needed to implement this architecture. The inertia of centralized, monolithic architecture has long prevailed and isn't easy to leave, but the "data mesh" architecture and its principles address significant issues plaguing data and analytics applications that many organizations face worldwide.
Quick Link
You may like
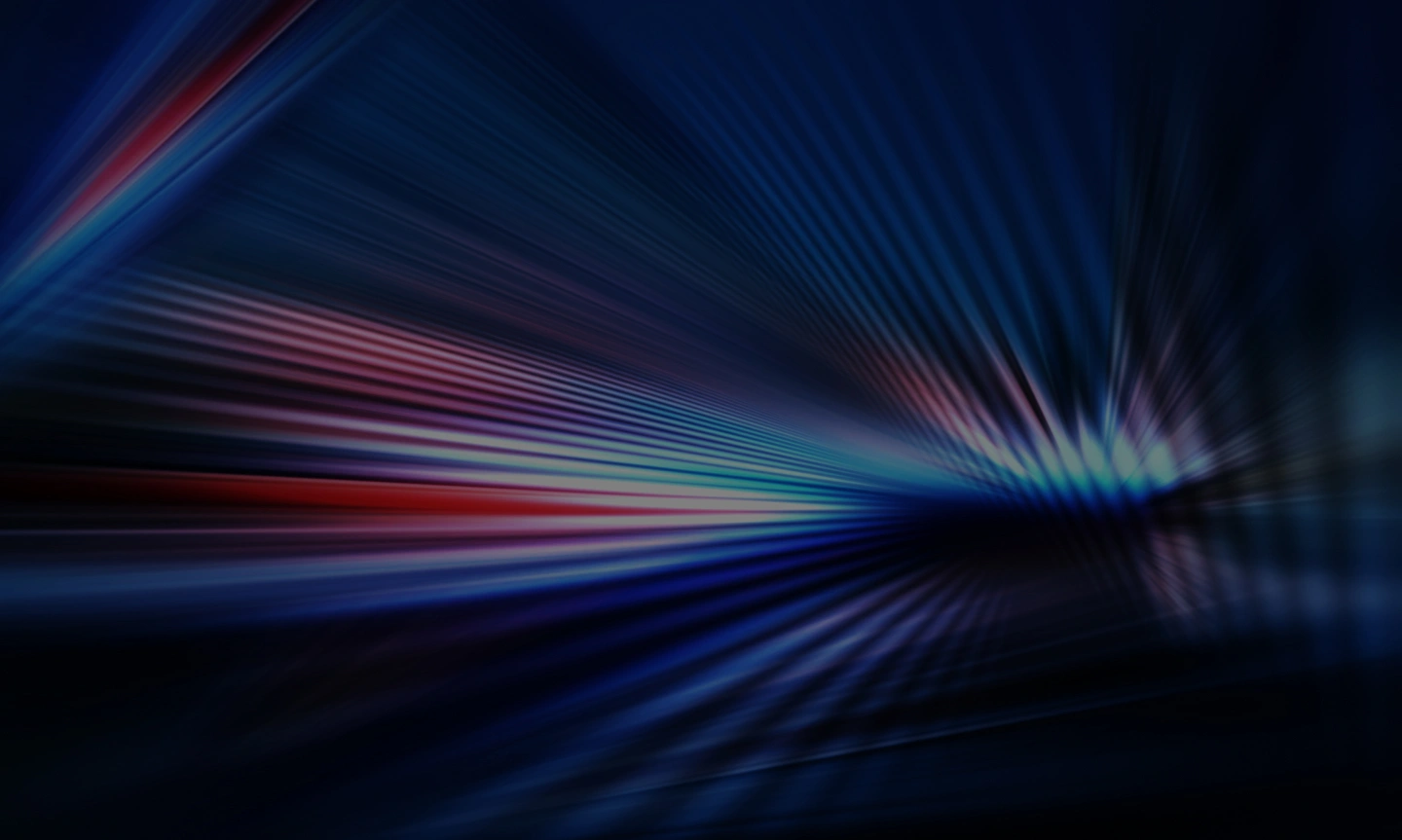
Data Warehouse vs. Data Lake vs. Data Mesh – Finding your way through data architectures
Helping organizations build a resilient data infrastructure.
READ MORE