Top 4 data & analytics architecture shifts for continuous innovation
October 05, 2022
Today, organizations are managing more data than ever before. Every organization has its data requirements that are varied by data sources, analytical needs, and governance requirements. Of course, legacy data architectures aren’t enough to handle today’s extensive data workloads.
So, over the past few years, forward-looking organizations have moved quickly towards new data infrastructure for delivering a seamless customer experience, innovation, and real-time decision-making.
However, this change has made data architectures more complex, sometimes hampering an organization’s ability to deliver timely innovations. Existing market competitiveness doesn’t allow such slowdowns. A new approach with robust data management is compulsory for organizations to keep a competitive edge.
Now is the time to step up and build a future-ready data architecture
Organizations are adopting new data management approaches for quicker deliveries, enhanced capabilities, and simplifying complex architectures. With all these new shifts, organizations can target almost all the data management phases like acquisitions, processing, storage, and analysis.
Here are the top shifts, modern organizations are undergoing to uplift their existing data architecture for managing their current data needs:
1. From complex data management to flexible data workloads
Predefined data models for advanced business intelligence need highly normalized schemas to minimize redundancy. While this approach may seem adaptable for advanced reporting, it is complex as well. It needs a lengthy development cycle that might affect the entire governance architecture.
Now, organizations are adopting schema-light architectures with denormalized data models for maximum performance. This leads to greater flexibility and powerful competitive advantage in the case of advanced analytics. This approach will enable agile data exploration and flexibility in data storage while reducing complexity. Some of the enabling concepts and components are Data Vault 2.0, Graph Database, and Azure Synapse Analytics.
2. On-premise cloud-based data platforms
Cloud is one of the most demanding technologies in recent years with the highest adoption rate compared to any other tool or technology. 67% of enterprise infrastructure is now cloud-based, whereas 48% of businesses choose to store their crucial data in the cloud.
Major cloud providers like AWS, Microsoft Azure, and GCP have revolutionized the way organizations source, display, and run data at scale. A few enablers are serverless data platforms like BigQuery and Amazon S3 and other containerized data solutions like Kubernetes. These concepts will enable organizations to decouple and automate the deployment of compute and additional data-storage systems.
3. Integration of real-time data processing tools
In recent years, the cost associated with real-time processing has decreased dramatically, making it the perfect choice for mainstream use. Real-time processing allows organizations to make decisions at the speed of business with increased agility and optimized costs. Companies can identify and act on short-term market changes.
Some of the best real-time processing and analytics solutions are Apache Kafka, Apache Flume, Apache Spark, and Apache Storm. These tools directly allow time for data analysis. For organizations that are in real-competitive businesses like finance, insurance, and travel, real-time streaming is a real competitive edge.
4. Adoption of domain-based data architecture
Data architects are now adopting a centralized data lake with a domain-driven design. This can be customized and fit for the purpose of improving time to market. In this approach, the data sets might reside on different platforms, with a product owner in each business domain. The architecture of a domain-based platform breaks enormous, monolithic enterprise data management into sub-divisions and business-specific domains like marketing, sales, and manufacturing.
In this case, data sets are an easily consumable way for users with domain and downstream data utilization. This decentralized, domain-based architecture distributes data and allows domain-specific teams to take ownership.
Robust data architecture is a must, not an option anymore
To become a fully functional data-centric organization, adopting a radically different, more advanced approach to data management is necessary. This will help organizations position themselves as agile and help them prepare for future demands.
At Systems, our teams are fully skilled in making your data platforms more agile and flexible by delivering robust data architecture. Our data platforms and analytical solutions are resilient enough to meet the competition. Get in touch with our experts.
Quick Link
You may like
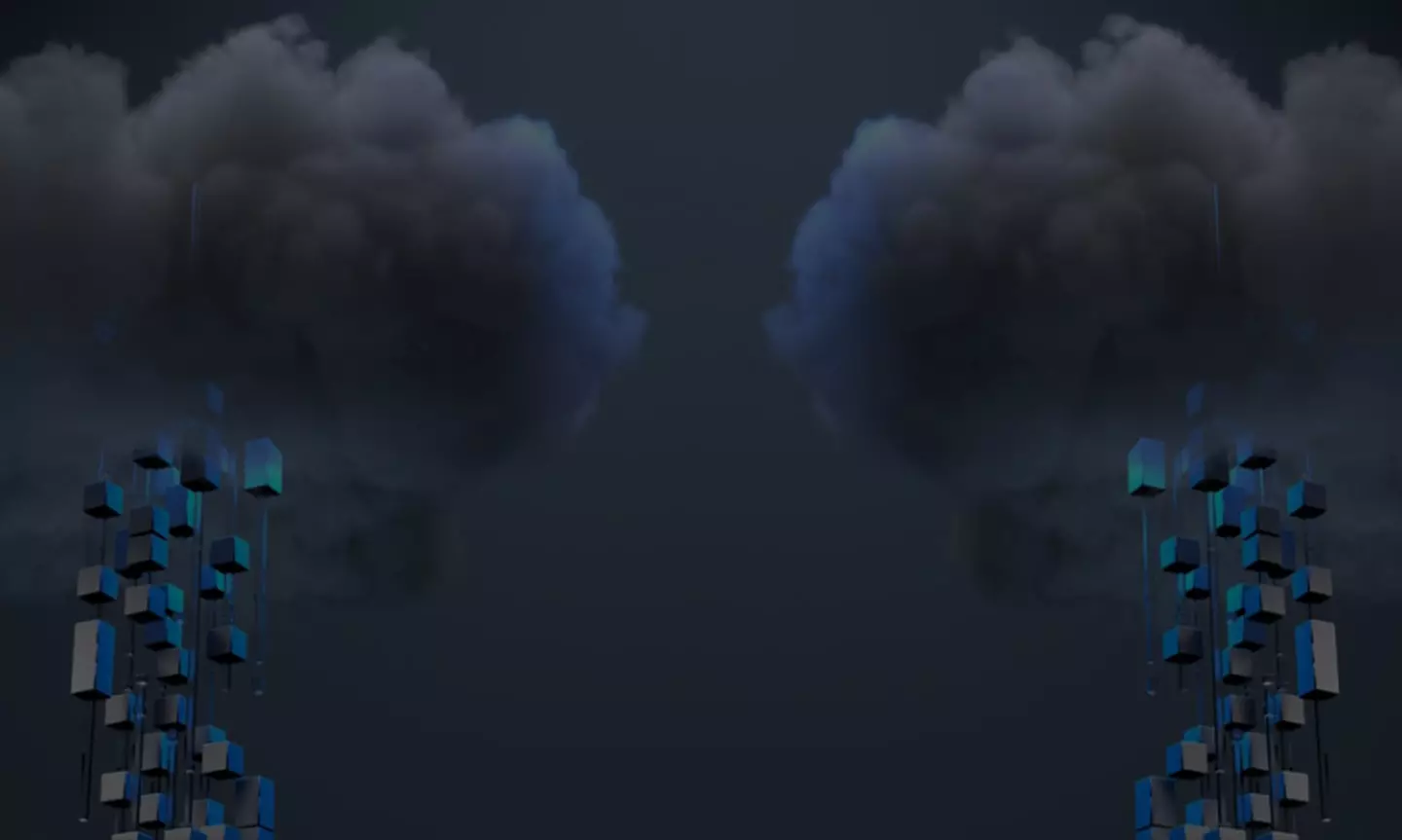
AWS vs. Azure – A brief guide on main differences
The brief guide highlights key differences between AWS and Azure, leaders in the cloud market, so
READ MOREHow can we help you?
Are you ready to push boundaries and explore new frontiers of innovation?