Enterprise data architecture - Best practices for building and scaling data analytics
September 21, 2023
Indeed, data analytics forms the core of Enterprise Data Architecture (EDA), serving as its beating heart. EDA not only establishes the infrastructure for data storage and integration but also focuses on enabling advanced analytics that extract valuable insights from the amassed data. By seamlessly integrating various data sources and optimizing their accessibility, EDA fuels data-driven decision-making processes, empowers predictive modelling, and ultimately propels the organization towards its strategic objectives.
What is enterprise data architecture?
Enterprise Data Architecture (EDA) serves as the blueprint for an organization's data management strategy, encompassing the design, integration, and organization of data assets. By aligning technology, processes, and business goals, EDA facilitates efficient data flow, enhances data quality, and supports informed decision-making – something that is crucial for your business.
The key role of data analytics
In today’s business landscape, data analytics stands as a cornerstone of success for enterprises. By meticulously analyzing vast and diverse datasets, businesses can extract meaningful insights that illuminate consumer behaviour, market trends, and operational efficiencies. The value of data analytics and enterprise data architecture lies in its capacity to unveil opportunities that would otherwise remain hidden, fostering innovation, competitive advantage, and sustainable growth in today's data-driven world.
- Data Collection: Data collection is a fundamental process within data analytics that involves gathering, acquiring, and assembling relevant data from various sources. This data serves as the raw material for analysis and is crucial for generating meaningful insights and making informed decisions.
- Data Storage: Data storage is a pivotal component of the data analytics process, as it involves securely housing and organizing the collected data for efficient analysis and retrieval. Effective data storage ensures that data is readily accessible, properly structured, and well-protected throughout the analytical lifecycle.
- Data Visualization: Data visualization is a pivotal component of the data analytics process, encompassing the representation of data through visual elements such as charts, graphs, maps, and dashboards. It serves as a means to transform complex and voluminous datasets into easily understandable and actionable insights.
Scaling up for unparalleled growth
When it comes to enterprise data warehouse architecture requires scaling up analytics within an organization with a strategic approach. Firstly, the organization needs to invest in robust infrastructure, either through expanding in-house hardware or adopting scalable cloud solutions, to handle increased data storage and processing demands. Developing a well-structured data architecture that integrates diverse data sources while ensuring data quality is essential. Implementing parallel processing and distributed computing techniques can optimize data analysis for speed and efficiency.
Advanced analytics tools should be selected to accommodate complex algorithms and machine learning models. Collaborative efforts between data analysts, engineers, scientists, and domain experts can help in devising comprehensive strategies. Regular monitoring and optimization of analytics processes, along with continuous skill development of the analytics team, will ensure that the organization is equipped to leverage data-driven insights effectively as it scales up its analytics endeavours.
Applying best practices for organizational data architecture
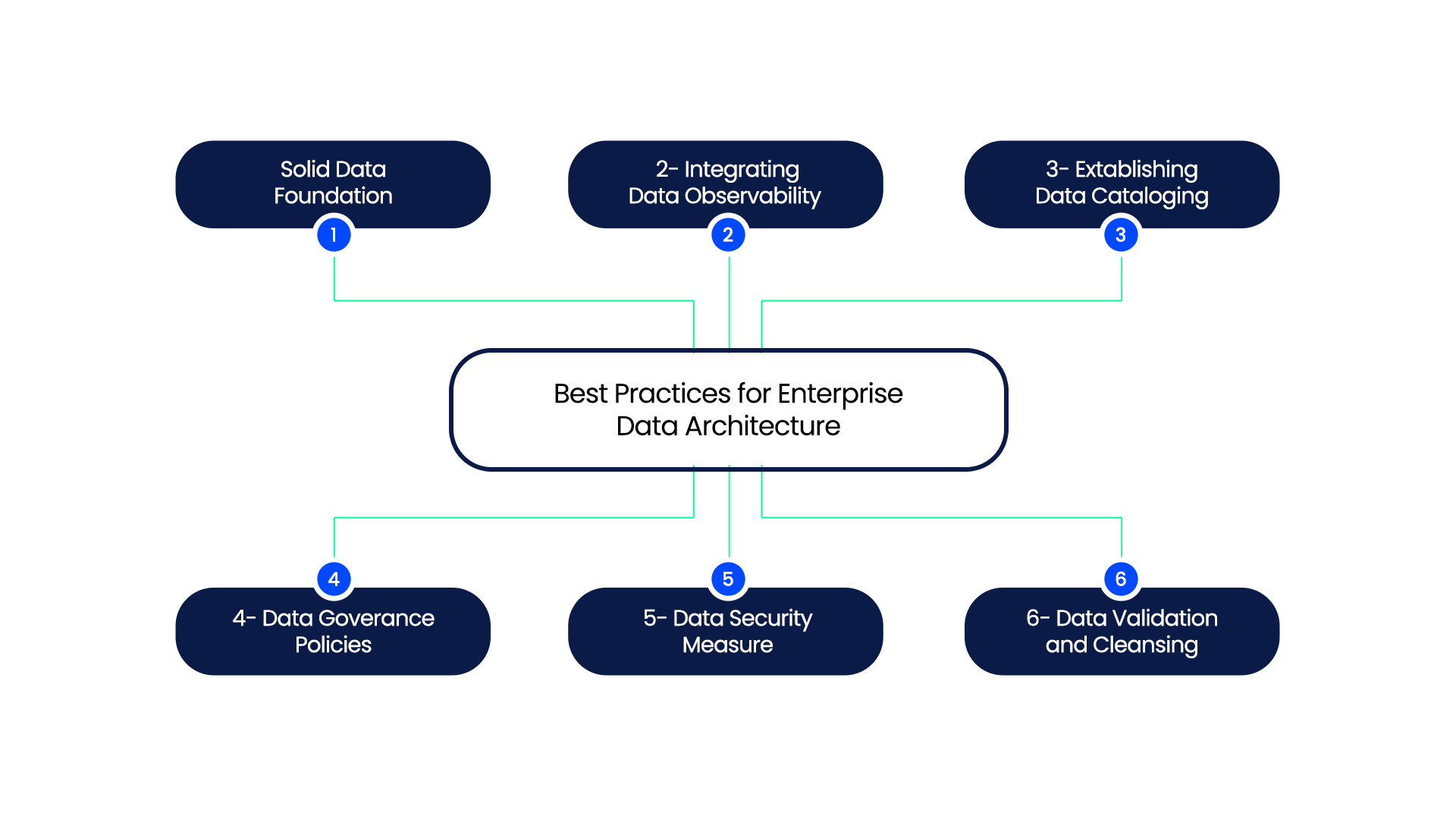
Implementing agile methodologies for data analytics workload
Implementing agile methodologies for data analytics is of paramount importance in today's rapidly changing business landscape. Agile approaches, originally developed for software development, have been adapted for data analytics to address the dynamic nature of data, evolving business requirements, and the need for quicker insights
The iterative nature of agile methodologies ensures that insights are continuously refined and improved based on feedback, leading to more accurate and relevant results. Ultimately, the implementation of agile methodologies in data analytics fosters a culture of collaboration, innovation, and data-driven decision-making, enabling organizations to stay competitive and thrive in a data-rich environment.
Integrating the right technology for data storage
The importance of selecting the right technology for your enterprise data warehousing architecture cannot be denied. The correct storage technology ensures that data is organized, accessible, and secure, facilitating informed decision-making and smooth business processes. Scalability is a key factor, allowing the infrastructure to adapt seamlessly as data volumes expand. Advanced security features, such as encryption and access controls, safeguard sensitive information against breaches and ensure compliance with regulations. Several technologies cater to diverse storage needs which are rational databases, NoSQL databases, and many other.
Understanding the needs for ETL & ML workload
The significance of choosing a relevant technology stack for ETL (Extract, Transform, Load) and machine learning (ML) workloads within a data warehouse cannot be overstated. The right ETL technology streamlines data extraction, transformation, and loading, enabling the seamless movement of data from diverse sources into the warehouse. ML algorithms can uncover patterns, predict trends, and automate decision-making processes, resulting in valuable business intelligence. In both cases, selecting technologies that align with the organization's data volume, complexity, scalability, and analytical goals is essential.
Scaling data analytics workloads is where the magic happens
Enterprise data architecture is where insights go beyond mere observations and become drivers of transformative change. Embracing scalable architectures, cloud technologies, and optimized data pipelines, businesses can rise to meet the challenges of exploding data volumes while preserving performance and accessibility.
The synergy of well-structured data, advanced analytics, and the power of machine learning brings forth a realm of possibilities. It empowers organizations to not only address current needs but also anticipate future trends, forecast outcomes, and pivot strategies. It's a journey of continuous learning and optimization, where data professionals and business stakeholders collaborate to unearth hidden insights and elevate decision-making to an art form.
Whether you're seeking seamless Data Warehouse implementation, cutting-edge data analytics expertise, or robust data management solutions, we've got you covered. Visit our data management and analytics page to explore how our expertise can reshape your data landscape and pave the way for a data-driven future.
Quick Link
You may like
How can we help you?
Are you ready to push boundaries and explore new frontiers of innovation?